News
Retail Insights Series: How AI is Giving Digital Marketers a New Edge on Visual Design
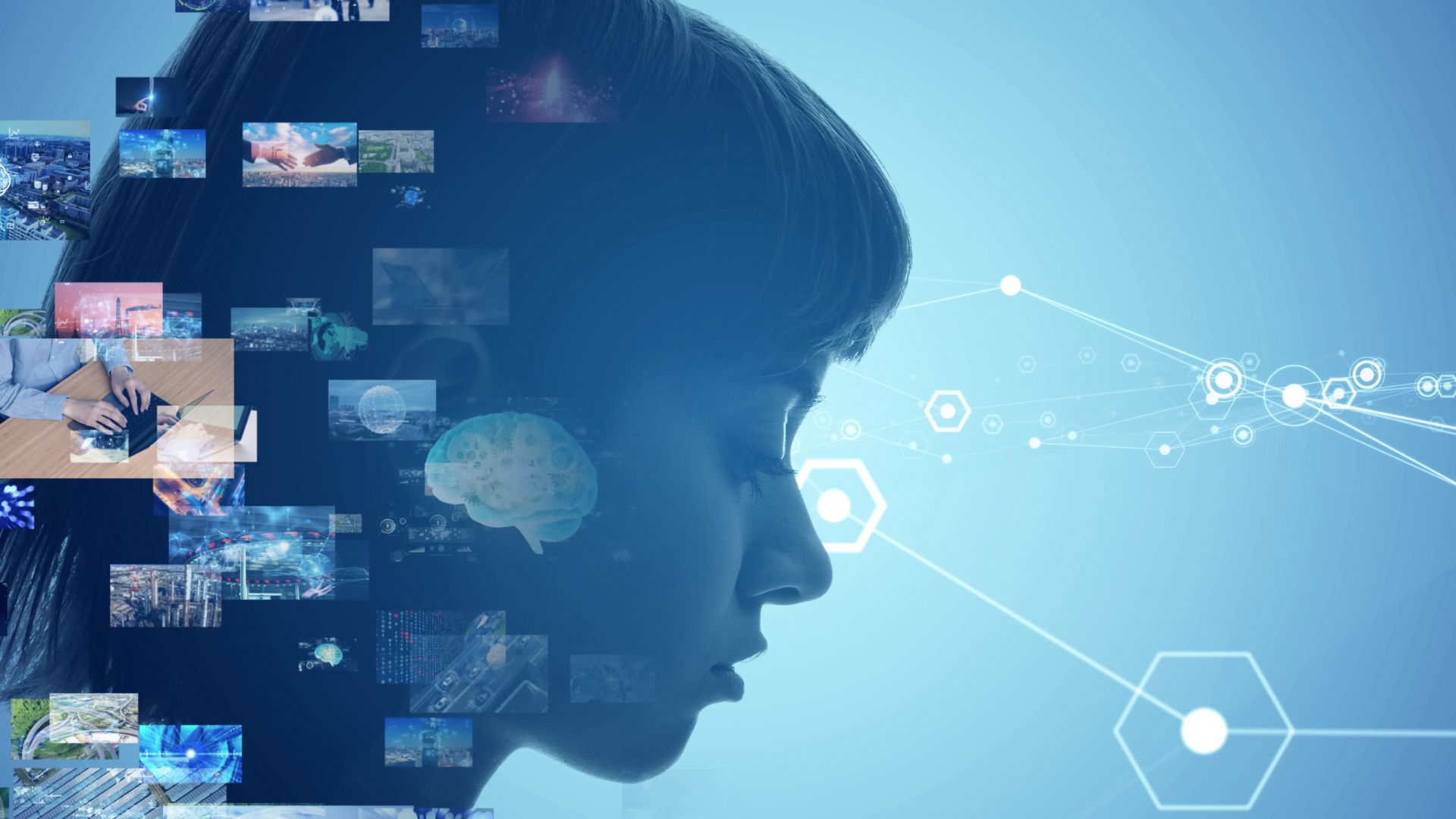
As the recent feats of the online chatbot ChatGPT have shown, AI has evolved to the point where it can work eye-popping wonders with text.
ChatGPT harnesses the power of what data scientists call Written Big Data. By retrieving and processing text-based information on the internet, the bot can answer questions on almost any topic, produce a script in the style of Jerry Seinfeld, and write college-quality essays.
Written Big Data also drives much of the work that tech-savvy marketers do in the retail space. For example, it enables them to generate insights from customer reviews and use that information to develop both their brand and their product.
But text forms only part of the vast amount of data now available to marketers.
As graphics, audio, and video become more popular communication modes, how can marketers derive insights from visual data?
That’s the question that fascinates Dr. Ethan Pancer, the Sobey Professor of Marketing in the Sobey School of Business at Saint Mary’s University. His research could help expand the role that visual data plays in the design of marketing materials.
“How do we drink from the fire hose?”
Dr. Pancer started his career in corporate marketing, so he understands the challenge of trying to capture and use a vast amount of marketing-related data. Today, he says, marketers are faced with an ongoing gush of data—but they’re taking advantage of only a trickle of it.
To date, text-based data mining has dominated marketing decision-making. Using textual analysis software, marketers can sift through thousands of words and sort them into various categories. They can also link word choices to specific qualities of the writing, such as authenticity and tone, and to the writer’s emotional state.
The kind of software required for such analysis, such as Linguistic Inquiry and Word Count, or LIWC (pronounced LIWC), has been around since the 1990s and is simple for users to operate. With a monthly subscription, any marketing analyst can quickly derive insights it once would have taken a linguistics scholar weeks or months to produce.
Let’s say, for instance, you’ve just conducted a two-week campaign on Facebook, promoting a new product line. If you collected all the Facebook comments into a txt file and ran them through LIWC, you’d get data about the kinds of words the comments contain (e.g., nouns, verbs, pronouns, etc.), the proportion of slang and formal word choices, and the emotional response associated with specific word choices.
That’s just for starters. Armed with the full battery of insights LIWC provides, you’d learn how to precisely shape the language of future campaigns to resonate with your target audience.
But you wouldn’t have a clue about how to design effective visuals for your next campaign because LIWC enables you to access just part of the massive, ever-flowing stream of data.
“How do we drink from the fire hose?” Dr. Pancer wants to know.
How do we advance past text-based data mining to collect and analyze multimodal data?
The Untapped Potential of Visual Big Data
LIWC examines a text the way a linguistic scholar would, rapidly applying the methods of traditional linguistic analysis at scale.
What if we could do something similar with visuals? What if software could scrutinize an image the way an expert designer would (but without human bias), rapidly and at scale?
By gathering and analyzing Visual Big Data, marketers could learn about the visual characteristics that resonate with their audience and use those insights to predict the success of marketing imagery.
In a recent study, Dr. Pancer and an international team of colleagues made significant progress toward that possibility. They used computer vision and other AI technologies to break down visuals into discrete bits of data.
Working with images from crowdfunding campaigns, they documented specific characteristics of images that marketers can monitor and manipulate. They also showed the potential of visual-based mining to help marketers predict the visual characteristics that result in persuasive images.
[insert image here to avoid stacking bold type on top of bold type]
Insights from a Crowdfunding Study
The study co-authored by Dr. Pancer examined more than 16,000 technology projects offered on the Kickstarter platform. The research team first identified specific characteristics that define an image, considering both technical aspects and the visual context. Then they developed computer vision software to scan images and detect those specific features. Finally, they used AI to identify factors that could help predict an image’s success in engaging the target audience.
“We wanted to find out what makes for a powerful image,” explains Dr. Pancer. “Is a picture really worth a thousand words?”
Dr. Pancer and his team trained their image-scanning software to recognize and measure eight different image characteristics:
- Number of pictures and videos
- Colorfulness
- Brightness
- Contrast
- Blur
- Number of faces
- Visual complexity
- Overall quality
If you’ve ever had to adjust the picture quality on a monitor or television, then such characteristics may sound familiar. But conventional marketing wisdom, as well as research into the role of image characteristics in marketing, has tended to focus on only the first point: the number of images. Dr. Pancer’s study showed, however, that more factors have the potential to help predict how well an image performs.
Besides expanding the number of visual features to consider, the software leveraged by Dr. Pancer and his colleagues made another breakthrough: it can help users understand visual context.
Scientists have long known that the image of an object is easiest to interpret when it’s depicted in the context of a scene that’s easy for the viewer to understand. For instance, Dr. Pancer likes to use the example of a novel juicer shown in a Kickstarter offering. If the juicer has an unusual form, it’s hard for an uninformed audience to determine its function from just a photo of the machine. But if the ad shows the juicer on a kitchen counter, next to a bowl half-full of oranges and a glass of orange juice, then the additional visual clues help the audience out. Putting scene and machine together, they quickly understand the juicer for what it is.
Pancer’s research suggests that AI may be able to reliably record and evaluate the visual context of an image. Armed with this intelligence, along with information about the image’s technical dimensions, a marketing analyst could then predict which version of an image would be most likely to succeed in the target market.
How You Can Start Working with Visual Data Today
Dr. Pancer stresses that it’s too early to derive best practices from his research. Context matters, and image characteristics that predict success on one platform may not translate perfectly onto another platform.
Down the road, research such as Dr. Pancer’s may result in a user-friendly tool that will enable marketers to instantly assess and modify an image for optimal performance. Such a tool would take the guesswork out of graphic design, making it as much a science as an art.
While you’re waiting for such a solution to develop, what can you do now to start tapping into more of that fire hose of marketing-related data? Dr. Pancer suggests three practical steps to try:
- Start tracking responses to visual elements. For example, run an A/B test of a blog post, changing only the header image, and measure audience engagement.
- Expand your design checklist. Go beyond counting the number of images in an ad or an in-store display. Make it part of your routine to consider technical categories of performance, such as colorfulness and contrast. For example, you might equip your social media team with a list of design criteria to use when evaluating templates for social posts or infographics.
- Pay attention to the visual context. Make sure you’re providing visual cues to help your target audience interpret key images. Such cues should be simple and familiar to the audience, anchoring the image in the world they know. For example, whenever you’re showing an image of a product, consider the visual frame around it. Does it hint at the physical setting where you’d expect to find the product? Does it enable the audience to picture themselves using it?
In the fast-growing field of AI, computer vision is one of the areas that’s developing the most rapidly. Stay tuned in to what’s happening with technology so you’ll be ready to take advantage of new tools for retail marketing as they emerge. Today’s exploratory research could well become tomorrow’s competitive advantage.
Check out Part 1 of January's edition of the Retail Insights Series as Dr. Pancer discusses "Turning Image Data Into Marketing Insights":